Synthetic data mimics real patient data, accurately models COVID-19 pandemic
National synthetic dataset boosts coronavirus research, helps prepare for future pandemics
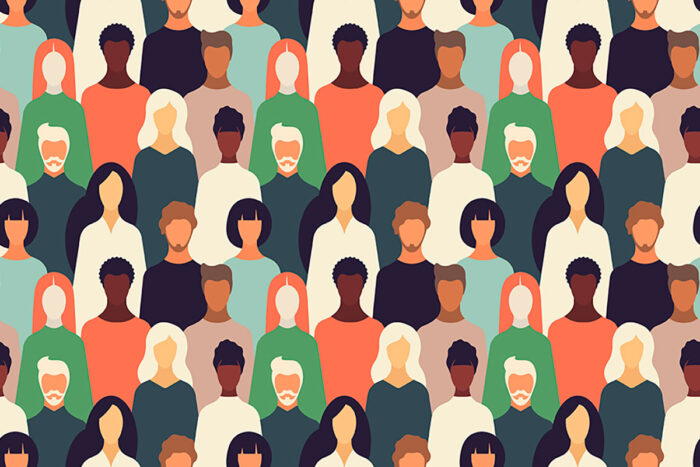
Research led by Washington University School of Medicine in St. Louis has demonstrated that analyzing synthetic data generated from real COVID-19 patients accurately replicates the results of the same analyses conducted on the real patient data. The School of Medicine has been a national leader in deploying and evaluating technology for the production of synthetic data, which is key for data-sharing collaborations across the country.
While caring for COVID-19 patients, health-care professionals across the country have amassed a treasure trove of information about SARS-CoV-2, its evolving variants such as delta and omicron, and their effects on the human body and public health. Such data, collected in patients’ electronic medical records, are vital for understanding the virus and developing treatments. But national data from medical records have been difficult for researchers to obtain because important processes that ensure patient privacy also slow down access to the data.
An initiative funded by the National Center for Advancing Translational Sciences of the National Institutes of Health (NIH) and co-led by Washington University School of Medicine in St. Louis has harnessed the tools of big data and advanced computation to provide researchers with massive quantities of synthetic data — modeled after real patient data — which is essential to understanding COVID-19 while also ensuring the protection of patient privacy and confidentiality.
Washington University School of Medicine, also part of the Center for Data to Health and the National COVID Cohort Collaborative (N3C), has been a national leader in deploying and evaluating technology for the production of synthetic data, which is key for data-sharing collaborations across the country.
The creation of synthetic data is the specialty of MDClone, a health-care informatics company that has worked with Washington University and other academic medical centers to help make synthetic data more widely accessible to researchers. Synthetic data are artificially generated, informed by actual patient data, but not directly derived from individual records, which substantially reduces the risk such data can be used to identify those persons. Instead of the traditional methods for concealing the identities of patients in datasets — such as deleting names, birthdates and other identifying information — the generation of synthetic data involves the production of a new set of simulated patients that, in aggregate, recreate the statistical characteristics of the real patients, such as measures of blood pressure, body mass index and kidney function. The real patients’ identities and privacy are protected because the simulated patients have no direct counterparts in the real data.
Research published in two studies led by Washington University School of Medicine has demonstrated that analyzing synthetic data generated from real COVID-19 patients accurately replicates the results of the same analyses conducted on the real patient data. Further, not only does the synthetic data accurately reflect the patient characteristics on a broad scale, the synthetic datasets accurately recreate the pandemic’s spread and impact over time and across densely tested geographic areas, allowing for investigations of the virus’ spread and impact at a population level.
One study is published in the Journal of the American Medical Informatics Association. The second study is available online in the Journal of Medical Internet Research.
“We’ve shown that we can build sophisticated predictions of what is going to happen in a population with a disease like COVID-19,” said co-author and principal investigator Philip Payne, PhD, the Janet and Bernard Becker Professor, chief data scientist and director of the Institute for Informatics at Washington University. “It is critical that we protect patients’ rights to privacy and confidentiality while also responding to the threat posed by COVID-19 in a timely manner. No single institution can address these needs alone. Through the unique capabilities afforded by the use of synthetic data, we are accelerating our efforts to diagnose, treat and, perhaps most importantly, prevent this disease while also demonstrating how we can more effectively respond to future public health emergencies.”
The use of synthetic data reduces the regulatory barriers that usually prevent the widespread sharing and integration of patient data across multiple organizations. Being able to share synthetic patient data allows researchers to analyze vast quantities of data from across the country rather than be limited to the data at their individual institutions. Researchers all over the world could apply for access to an institution’s synthetic data to conduct their own studies. This capability increases the scale and efficiency of such research while also reducing potential biases in ensuing findings.
To date, the N3C synthetic dataset includes data from 72 institutions across the country and contains records representing 13 million patients. Of those, about 5 million patients had a positive COVID-19 test. With the massive synthetic datasets generated from this resource, researchers can look for patterns in the data that would not emerge with smaller sample sizes. Using state-of-the-art informatics and data science tools, such as pattern recognition and machine learning techniques, the data could identify criteria that predict which patients are at highest risk of needing intensive care or ventilators. It also could help pinpoint patterns in treatment strategies to see if drugs that a COVID-19 patient is already taking for a different condition — say a blood thinner for heart disease — might be protective or harmful compared with patients not taking that drug.
The first paper demonstrated that the synthetic data accurately reproduced the demographics and the clinical characteristics of the patients in the initial N3C dataset. Synthetic data also could be used to accurately predict the risk of hospital admission or readmission for patients diagnosed with COVID-19. In addition, population-level epidemic curves, such as number of cases per day, number of hospitalizations and deaths per day and seven-day rolling averages of positive cases over specific time frames also were accurately reproduced by the synthetic data. The second paper included a deeper analysis of the epidemic curves in subsets of populations living in specific ZIP codes. In this case, too, the synthetic dataset accurately mimicked the spread of the pandemic across different geographic regions as long as those regions were densely tested for COVID-19. Analyses in the second paper using small sample sizes or populations were less capable of reproducing results in the real dataset.
“Being able to look at specific ZIP codes is extremely important in analyzing a pandemic, since social determinants of health vary by where a patient lives,” said Adam Wilcox, PhD, a professor of medicine and senior author of both studies. “We know that social determinants of health — such as access to health care, education and economic stability — are related to COVID-19 transmission and outcomes. This analysis shows that we can use synthetic data to study different dynamics of a pandemic, including how the pandemic changes over time and across geographic area. These papers represent a really thorough investigation of the capabilities of synthetic data for pandemic modeling.”
According to the researchers, synthetic data is best at representing what is happening at a broad population level but is not as good at analyzing outliers. Outliers involving small numbers of patients with combinations of rare characteristics or situations in which a geographic region contains very few people, such as in rural ZIP codes, are intentionally excluded from synthetic datasets to further protect the privacy of individuals who may fall into those categories. However, in general, it is difficult for data analyses to be representative when looking at small numbers, so this challenge is not unique to synthetic data.
“We are continuing to test the boundaries of what we can do with synthetic data, so we understand the best uses of this type of data and also the situations when we need to go back to the original data,” said Randi Foraker, PhD, a professor of medicine and the first author of the second study and a co-author on the first study. “There are situations where synthetic data may not be as accurate as the original data, and we need to know what those are to be able to select the best methods possible for analyzing a particular dataset.”
On a broad scale, the researchers said the data allows for the prediction of future hot spots of COVID-19, so those areas can prepare for and potentially head off a worst-case scenario. The synthetic data systems now in place also will help researchers respond faster to a future pandemic. Payne compares it to weather forecasting.
“We’re trying to build the hurricane-track equivalent for pandemics, using large amounts of data,” Payne said. “When weather forecasting works, it’s because they have a lot of prior data to learn from, and they’re able to apply that to what they’re observing now. Then they create a variety of different models predicting future scenarios — in this case, potential paths of the hurricane — and the probabilities of each. We’re building tools to do exactly the same thing but for infectious disease pandemics.”